Admin مدير المنتدى
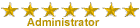
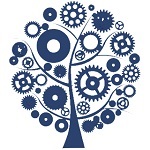
عدد المساهمات : 18996 التقييم : 35494 تاريخ التسجيل : 01/07/2009 الدولة : مصر العمل : مدير منتدى هندسة الإنتاج والتصميم الميكانيكى
 | موضوع: كتاب Assembly Line Design الأحد 11 أكتوبر 2020, 10:11 pm | |
| 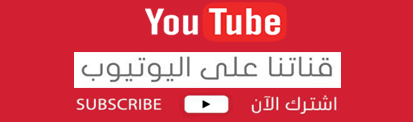
أخوانى فى الله أحضرت لكم كتاب Assembly Line Design The Balancing of Mixed-Model Hybrid Assembly Lines with Genetic Algorithms With 95 Figures Brahim Rekiek and Alain Delchambre
 و المحتوى كما يلي :
Contents Part I Assembly Line Design Problems 1 Designing Assembly Lines . 3 1.1 Introduction 3 1.2 Assembly Line Design 3 1.3 Designing or Optimising? . 5 1.4 Layout of the Book 6 2 Design Approaches 7 2.1 Introduction 7 2.2 Why the Design is Difficult? 8 2.3 Design and Search Approaches 8 2.4 The Gap Between Theory and Practice . 8 2.4.1 Input Data . 9 2.4.2 Multiple Objective Problem 9 2.4.3 Variability 9 2.4.4 Scheduling 9 2.4.5 Layout . 10 2.5 About the Quality of a Design 10 2.6 Assembly Line Design Evolution . 10 3 Assembly Line: History and Formulation 13 3.1 Introduction 13 3.2 Evolution of Today’s Manufacturing Issues 13 3.2.1 First Metals 13 3.2.2 Carpenters and Smiths . 13 3.2.3 Cottage Industries . 14 3.2.4 Factory System . 14 3.2.5 Mass Production 14 3.2.6 Computers in Manufacturing . 15 3.3 Assembly Line Systems 15 xiiixiv Contents 3.4 Notation and Definitions . 16 3.5 Assembly Line Balancing Problems 19 3.5.1 Assembly Line Models . 19 3.5.2 Variability of Tasks Process Time . 20 3.5.3 Line Configuration 21 3.5.4 Additional Constraints . 23 3.5.5 Assembly Line Design Problems . 25 3.6 Why is the Balancing Problem Hard to Solve? 27 Part II Evolutionary Combinatorial Optimisation 4 Evolutionary Combinatorial Optimisation 31 4.1 Introduction 31 4.2 System Organisation . 31 4.3 How Do Genetic Algorithms Work? 32 4.3.1 Representation 33 4.3.2 Initialisation of the Population 34 4.3.3 Sampling Mechanism 35 4.3.4 Genetic Operators . 36 4.4 Landscapes and Fitness 38 4.5 Population . 38 4.6 Simple... but it Works! . 38 5 Multiple Objective Grouping Genetic Algorithm . 39 5.1 Introduction 39 5.2 Multiple Objective Optimisation 39 5.3 The State of the Art . 40 5.3.1 The Use of Aggregating Functions . 41 5.3.2 Non-Pareto Approaches 41 5.3.3 Pareto-based Approaches . 42 5.3.4 Preferences and Local Search Methods . 42 5.3.5 Constrained Problems 43 5.4 Grouping Problems and the Grouping Genetic Algorithm . 44 5.4.1 Encoding Scheme 44 5.4.2 Crossover Operator 45 5.4.3 Mutation Operator 46 5.4.4 Inversion Operator 46 5.5 Multiple Objective Grouping Genetic Algorithm . 46 5.5.1 Control Strategy 47 5.5.2 Individual Construction Algorithm 48 5.5.3 Overall Architecture of the Evolutionary Method 48 5.5.4 Branching on Populations 49 5.6 The Detailed Example . 51Contents xv Part III Assembly Line Layout 6 Equal Piles for Assembly Line Balancing 59 6.1 Introduction 59 6.2 The State of the Art . 59 6.2.1 Exact Methods 59 6.2.2 Approximated Methods 61 6.3 Equal Piles for Assembly Line Balancing . 62 6.3.1 Motivation and Inspiration From Nature . 63 6.3.2 Input Data . 64 6.3.3 Customising the Grouping Genetic Algorithm to the Equal Piles Assembly Line Problem 64 6.3.4 Experimental Results 69 6.4 Extension to Multi-product Assembly Line . 71 6.4.1 Multiple Objective Problem 71 6.4.2 Overall Architecture . 72 7 The Resource Planning for Assembly Line . 77 7.1 Introduction 77 7.2 The State of the Art . 78 7.3 Dealing with Real-world Hybrid Assembly Line Design . 79 7.3.1 Cost . 79 7.3.2 Process Time . 80 7.3.3 Availability . 82 7.3.4 Station Space . 83 7.3.5 Incompatibilities Among Several Types of Equipment 84 7.4 Input Data . 84 7.5 Overall Method . 85 7.5.1 Distributing Tasks Among Stations 85 7.5.2 Selecting Equipment . 86 7.5.3 Heuristics 89 7.5.4 Dealing with a Multi-product Assembly Line 90 7.5.5 Complying with Hard Constraints . 91 7.6 Application of the Method 92 8 Balance for Operation . 93 8.1 Introduction 93 8.2 Multi-product Assembly Line . 93 8.3 The State of the Art . 94 8.3.1 Classical Methods . 94 8.4 Heuristics 95 8.5 Ordering Genetic Algorithm 95 8.5.1 Algorithm 95 8.5.2 Heuristics 97xvi Contents 8.6 Balance for Operation Concept 99 8.6.1 Non-fixed Number of Stations . 100 8.6.2 Fixed Number of Stations 102 Part IV The Integrated Method 9 Evolving to Integrate Logical and Physical Layout of Assembly Lines 105 9.1 Introduction 105 9.2 The State of the Art . 105 9.3 Assembly Line Design 106 9.4 Integrated Approach . 106 9.4.1 Development of the Interactive Method 108 9.4.2 Global Search Phase . 115 9.5 Application . 116 10 Concurrent Approach to Design Assembly Lines . 121 10.1 Introduction 121 10.2 Concurrent Approach 121 10.3 Assembly Line Design 122 10.3.1 Data Preparation Phase 123 10.3.2 Optimisation Phase 124 10.3.3 Mapping Phase . 124 10.4 Case Studies 124 10.4.1 Assembly Line Balancing Application: Outboard Motor 125 10.4.2 Resource Planning Application: Car Alternator . 128 11 A Real-world Example Optimised by the OptiLine Software137 12 Conclusions and Future Work . 145 12.1 We Attained.. . 145 12.2 Tendencies and Orientations 145 12.3 Data Collection . 146 12.4 Model Formulation 146 12.5 Validation and Output Analysis . 146 12.6 The Proposed Approach 147 References . 149 Index 159List of Abbreviations AI Artificial intelligence AL Assembly line ALB Assembly line balancing ALD Assembly line design B&B Branch and bound B&C Branch and cut BD Balance delay BFO Balance for operation BPP Bin packing problem CAD Computer aided-design CE Concurrent engineering CISAL Outils d’aide `a la conception interactive des produits et de leur ligne d’assemblage CM Cellular manufacturing COP Combinatorial optimisation problem CS Capacity supply DFA Design for assembly DM Decision maker DP Dynamic programming E Line efficiency EPALP Equal piles for assembly line problem ES Evolutionary strategies FABLE Fast algorithm for balancing line effectively FFD First fit decreasing FG Functional group GA Genetic algorithm GC Goal chasing method GGA Grouping genetic algorithm GT Group technology HAL Hybrid assembly line I Line idle time xviixviii List of Abbreviations IB Imbalance ICA Individual construction algorithm JIT Just in time LL Logical layout LP Linear programming MAL Manual assembly line MCDA Multi-criteria decision-aid ML Model launching MOALBP Multiple objective ALBP MOB-ES Multiple objective evolution strategy MOEA Multiple objective evolutionary algorithm MOGLS Multiple objective genetic local search MOGA Multiple objective genetic algorithm MOGGA Multiple objective grouping genetic algorithm MOP Multiple objective problem MPAL Multi product assembly line MWkCALB Multiple workcentres ALBP NPGA Niched pareto genetic algorithm NSGA Non-dominated sorting genetic algorithm OGA Ordering genetic algorithm OMT Operating modes and techniques OV Ordering variants OX Order crossover PBX Position based crossover PG Precedence graph PL Physical layout PMX Partially mapped crossover PROMETHEE Preference ranking organisation Method for Enrichment evaluations PSGA Problem space genetic algorithm RD-MOGLS Random directions multiple objective genetic local search RP Resource planning RPW Ranked positional weight RRPW Reversed ranked positional weight RWS Roulette wheel selection SA Simulated annealing SALBP Simple ALBP SMCT Scheduling method choice tool SPAL Simple assembly line balancing SPEA Strength pareto evolutionary algorithm ST Station time SX Smoothness index (SX) TALB Tree assembly line balancing TS Tabu search TVR Time variability ratio VEGA Schaffer’s vector evaluated GA Index AL, see Assembly lines Artificial intelligence, 49 Assembly lines, 3–6, 10, 13, 15–21, 25, 26, 44, 71, 73, 79, 90, 95, 97, 105, 106, 121, 122, 147 Assembly lines balancing, 4, 6, 13, 16, 19, 20, 25, 26, 48, 59, 62, 121 Assembly lines design, 3, 9, 10, 25 B&C, see Branch and cut Balance for operation, 6, 9, 93, 99, 121, 122 Batch, 62, 97, 110, 145 Batch production, 11, 20 BF, 62 BFO, see Balance for operation Bin packing problem, 27, 62 Boundary stones algorithms, 65, 66, 68, 145 BPP, see Bin packing problem Branch and bound, 59, 60 Branch and cut, 48, 85–87 Branching on population, 49 Capacity supply, 17 Cellular manufacturing, 105 Clustering, 67, 105, 107, 108, 110, 111 Combinatorial optimisation problems, 6, 60 Computer-aided design, 10, 145, 146 Concurrent engineering, 4, 7, 8, 100 Constraint, 23, 24, 60, 85, 87, 90, 110 Cost function, 69, 74, 115 Crossover, 32, 33, 35–38, 44, 45, 67, 96 Cycle time, 67–70, 73, 74, 79, 86, 88, 92, 98–100, 102, 107, 110, 113, 114, 116, 118, 121, 123, 131, 133, 141 Decision maker, 39, 46, 51, 135 Design for assembly, 3 Deterministic time, 20 DFA, see Design for assembly Diversity, 35, 37, 38, 41, 42, 56 DP, see Dynamic programming Dynamic programming, 59, 60 Dynamic time, 21 Elitist model, 35 Equal piles, 6, 26, 48, 59, 62, 63, 78, 85, 116, 126 Evolutionary strategies, 41 Exact methods, 59 Feasibility, 34, 78 FF, 62 FFD, see First fit descending FG, see Functional groups First fit descending, 62, 63 Fixed operations on stations, 23 Functional groups, 79, 81, 82 GA, see Genetic algorithms Genetic algorithms, 34, 36, 70, 78, 95, 148 Genetic operator, 34, 36, 38 Graph search, 61 Group technology, 15, 105 Grouping, 72, 111, 145 159160 Index Grouping GA, 62–64, 78, 91, 92, 145 Grouping genetic algorithm, 45 HAL, see Hybrid assembly line Heuristics, 61, 78, 89, 95, 97, 115, 124, 145 Hidden time, 21, 80, 81, 84, 91 Hybrid AL, 79 Hybrid assembly line, 4, 89 ICA, 48, see Individual construction algorithm Idle time, 18, 59, 89, 97, 106 Imbalance, 17, 74 Individual construction algorithm, 49, 85 Input data, 73, 88, 115, 123, 146 Integrated approach, 105, 106, 121 Inversion, 36, 37, 44, 46, 96 JIT, 21 Just in time, 21 Line configuration, 21, 61 Line efficiency, 18, 26, 59 Linear programming, 60 Local search, 40, 42, 43 Logical layout, 105, 108, 111, 121, 124, 126 Logical line layout, 4 Max peak time, 79, 100, 102 MCDA, see Multi criteria decision aid Metaheuristic, 61, 62, 69 Mixed production, 11, 146 Model launching, 93, 94 MOGA, see Multiple objective GA Multi criteria decision aid, 39, 40, 46–48, 74, 86, 148 Multi objectives, 71, 132, 145, 148 Multiple objective GA, 39, 42, 43, 46 Mutation, 32, 35, 36, 44, 48, 56, 67, 96 Natural evolution, 32, 33 OGA, 93, 95 OptiLine, 6, 138, 141 Optimisation, 60, 78, 105, 108, 111, 123, 124, 133, 135 Order crossover, 96 Ordering variants, 25, 26, 93–96 OV, see Ordering variants Parallel lines, 21 Parallel stations, 4, 21 Partially mapped crossover, 96 Physical layout, 4, 6, 10, 25, 83, 105, 107, 124, 126 Population, 32, 35, 38, 41, 42, 46, 47, 49, 50, 64, 67, 72, 100 Precedence constraints, 3, 4, 16, 17, 25, 61–63, 67, 68, 78, 79, 84, 89, 92, 100, 108, 110, 115–117, 123, 125–127, 129–131, 142 Precedence graph, 64 Process time, 7, 10, 17, 18, 20, 21, 25, 61, 63, 80, 85, 88, 90, 98, 102, 113, 114, 116, 125, 129, 132, 145 Product analysis, 3, 7 PROMETHEE II, 46, 48, 49, 72, 74, 85, 86, 88, 90, 145 Resource planning, 4, 6, 8, 25, 26, 48, 90, 121, 124, 128 Robot, 3, 4, 16, 20, 24, 97, 124 RP, see Resource planning Scheduling, 9, 20, 93, 94, 99, 142, 145 Serial lines, 21 Simulated annealing, 37 Single product line, 19 Smoothness index, 19 Stochastic time, 20 Stochastic universal sampling, 36 Tabu search, 37 TALB, 95 Throughput, 3, 18, 20, 63, 93, 110 Time interval, 18 Time variability ratio, 18 Variant, 9, 11, 19, 72, 79, 90, 94, 107, 110 Vector evaluated genetic algorithm, 41 VEGA, see Vector evaluated genetic algorithm, 43 Work content, 18 Workcentre
كلمة سر فك الضغط : books-world.net The Unzip Password : books-world.net أتمنى أن تستفيدوا من محتوى الموضوع وأن ينال إعجابكم رابط من موقع عالم الكتب لتنزيل كتاب Assembly Line Design رابط مباشر لتنزيل كتاب Assembly Line Design 
|
|